Short version
Use one of the following files to determine the Android version of a digital forensic extraction that is lacking the \system\build.prop file:
- \data\system\usagestats\0\version
- \data\system_ce\0\usagestats\version
Long version
Most automated tools that parse Android full file system extractions depend on the /system/build.prop file to determine the Android version among other device identifiers. Due to how variable are Android implementations as well as data extracting software a build.prop file might not be available. Is there a way to determine the Android version of an extraction by only looking at the userdata directory? The answer is yes. This was useful to me since some of my digital forensics tooling for Android extractions would benefit from programmatically identifying the Android version when a build.props file is not available.
 |
by: https://gs.statcounter.com/android-version-market-share/mobile-tablet/worldwide |
UsagestatsOne of the ways Android devices keep track of application activity is by registering events in the usagestats directory. Depending on the Android version these can be kept in XML or protobuf format. These can be found in the following locations depending on your Android version:
- \data\system\usagestats\0\
- \data\system_ce\0\usagestats\
For a quick explanation on usagestats and their applicability see here: https://abrignoni.blogspot.com/2019/02/android-usagestats-xml-parser.html
To parse usagestats data you can use ALEAPP (Android Logs Events And Protobuf Parser) here: https://github.com/abrignoni/ALEAPP
The usagestats folder contains a plain text file called version. It is usually composed of two lines. The first one being a number and the second one a series of alphanumeric values separated by semicolons.
 |
Usagestats/version file from a Samsung SM-G960U |
The filename clearly indicated that the content had to be versioning related. Since the build.props file is well understood and documented I made a comparison between the too to try and determine the provenance of the version's file content if possible.
Notice in the following image the side by side comparison and color coding of similar values of files extracted from a Samsung device.
From the version file's second line we can determine the following:
- First items = Version release = Android version
- Second item = Codename = Fingerprint
- Third item = Incremental build version
- Fourth item = CRC = Country Specific Code
It is of note that Pixel devices have less values within the version file. There is no CRC and no final value. Still the Android version was the same as the one located in the build.props file. This was true across all sample extractions I was able to check.
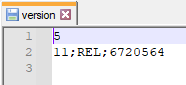 |
Version file from a Pixel phone |
What about the number on the first line? Through the process of testing the values on the second line a pattern appeared for the number in the first line. It is as follows:
- 3 = Android 8, 9
- 4 = Android 10
- 5 = Android 11
Jessica Hyde (
@B1N2H3X) suggested I take into consideration how the file would look, if anything, after an operating system upgrade. Great point! Thanks to Josh Hickman (
@josh_hickman1) that was really easy to do. He has well documented test Android images for community use and testing. By looking at the values within the version file on his Android 10 extraction and then the values on the upgraded Android 11 image I was able to determine that an update would produce an additional file in the directory where version resides called migrated.
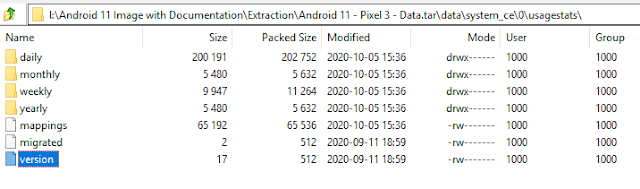 |
The migrated file. |
The migrated file contains the number in the first line of the version file previous to the upgrade. After the upgrade the version file contains the numbers that are consistent with the current version. The next image is the value contained within the migrated file in the Android 10 extraction.
 |
Migrated file with a value of 4 |
Now compare it with the values within the version file in the same Android 11 extraction.
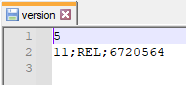 |
Version file with a value of 5 |
This behaviour was also confirmed by Carlos Eduardo (
@GalloDu) with his own upgraded device data.
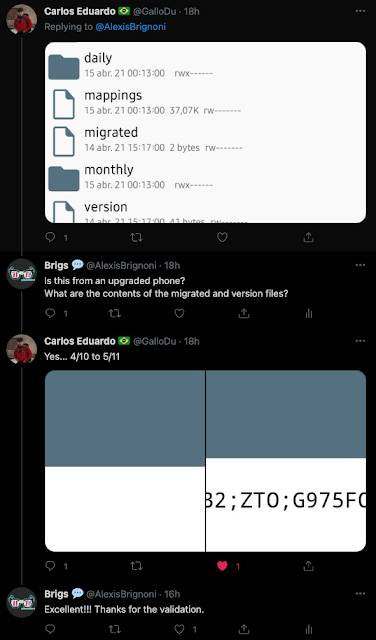 |
Confirmation of migrated & version relationship |
Based on this behaviour it follows that the presence of a migrated file indicates a major operating system upgrade. By comparing the contents of the migrated and version files the analys can determine from and to what version the device was upgraded to.
Pending work
For this analysis I only had access to Samsung and Pixel files. It would be of use if migrated and version files from other vendors (LG, OnePlus, etc...) are shared to see how they might defer and/or what additional data they might provide if any.
Implementation
I have made a parser for the version file within ALEAPP. The script will identify and use Android version number contained in either the build.props or version file for reporting and artifact purposes. To quickly view the data press the device details tab at ALEAPP's report home page.
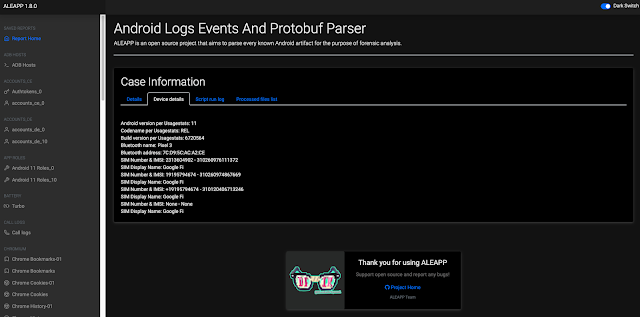 |
Device details tab with Android version data |
As always, I can be reached on twitter @AlexisBrignoni and email 4n6[at]abrignoni[dot]com.
-Brigs